A Milestone Dataset on the Road to Self-Driving Cars Proves Highly Popular
The idea of an autonomous vehicle – i.e., a self-driving car – isn’t particularly new. Leonardo da Vinci had some ideas he wrote down in 1478, and actual prototypes appeared in controlled settings even before World War II. But only in the last couple of years has the prospect of widespread consumer use been within reach.
So a decade ago, when four researchers created a dataset built around key aspects of autonomous vehicles, it turned out they were neither ahead of their time nor behind it, but of it. The work describing the KITTI dataset (reflecting its development by the Karlsruhe Institute of Technology and Toyota Technological Institute at Chicago) is the most-cited paper published in a Sage journal in 2013, and so received a Sage 10-Year Impact Award. “Vision meets robotics: The KITTI dataset,” by Andreas Geiger, then of the Karlsruhe Institute of Technology and Max Planck Institute for Intelligent Systems, Philip Lenz and Christoph Stiller, both at the Department of Measurement and Control Systems at the Karlsruhe Institute of Technology, and Raquel Urtasun of Toyota Technological Institute, and published in The International Journal of Robotics Research, has received 4,721 citations according to Web of Science, and 5,438 based on the methods used by Crossref. The paper describes the researchers’ recording platform and sensors mounted on a Volkswagen station wagon, the data format they used and the utilities they provided for future users of the dataset.
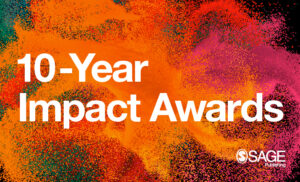
This is the fifth year that Sage, the parent of Social Science Space, has awarded 10-Year Impact Awards to the top three most-cited papers of the past decade. As Sage’s president of global publishing, Ziyad Marar, explains, “The impact of academic research, especially in the social and behavioral sciences, often goes beyond the standard two-year citation window. These awards extend that period to 10 years, recognizing work with a deep and lasting impact that might be overlooked in the short term.
We asked the lead author of the article, Andreas Geiger, now head of the Autonomous Vision Group at the University of Tübingen, to reflect on the paper, KITTI, and the nexus of robotics, computer vision and machine learning.
In your estimation, what in your research—and obviously the published paper—is it that has inspired others or that they have glommed onto?
In 2011, when we started developing the KITTI dataset, there existed no other big datasets and in particular no evaluation benchmarks for independently testing perception algorithms for self-driving. Different research papers used different datasets for evaluation, different metrics, and different baselines. As a consequence, existing methods could not be fairly evaluated and compared against, and the state-of-the-art could not be determined. The KITTI dataset and evaluations have changed this and led to significant improvements across various perception tasks such as 3D reconstruction, object recognition, and vehicle localization. These improvements came about for two reasons: First, self-driving has been popularized as a task in the computer vision community and many people have started working on this problem. In fact, today we have over 70.000 registered accounts on our server.
Second, through fair benchmarking, the state-of-the-art could always be determined, leading to much more rapid progress than was possible before. In addition, the move to open access and open source in our community of course helped with this acceleration. Today, KITTI is recognized as the seminal dataset and benchmark in computer vision for self-driving and many other datasets and benchmarks have followed, including the popular Waymo and the nuScenes datasets.
In the decade since your paper, the idea of self-driving cars have moved into the public consciousness and onto city streets. Has the progress been faster or slower than you might have expected in 2013? How important has having a well-curated dataset or a leaderboard been to that progress?
On one hand, we have made tremendous progress and I would not have believed that we have robo taxis on the streets today. On the other hand, these systems are still not fully ripe yet, as they can only be deployed with a fall-back driver or in geofenced situations using teleoperation for handling corner cases. It is just a very hard problem. Human driving results in 1 fatality every 100 million miles. This is a very hard error rate to beat. And we have to beat it by 10x or 100x to be trustworthy. It is hard to quantify exactly how KITTI has supported this progress as research builds on top of past research and industrial research is not public. But from what we see, KITTI has been a huge accelerator, improving accuracy for some tasks by over 10x, and popularizing self-driving research in computer vision and robotics.
We have also seen that many industrial efforts are built on research results from the community. Hence, I do believe that KITTI had a significant impact on both academic research as well as industrial progress in self-driving.
What, if anything, would you have done differently in the paper (or underlying research) if you were to go back in time and do it again?
Good question. I think we severely underestimated the time it took to get the full pipeline working, from data acquisition over sensor calibration to annotation, curation and publication as well as development of the evaluation server. Today, much more mature and advanced systems are available (both hardware and software) that can take over large portions of this work, but these haven’t been available in 2011. Also, every dataset and benchmark that becomes popular bears the risk that researchers overfit to that particular dataset. If the whole community uses it, reviewers will ask authors to benchmark on it. However, at some point, benchmarks become saturated and better datasets exist and the community should move on. But this transition is sometimes hard. Also, some of the calibrations and annotations we did were not accurate enough to evaluate state-of-the-art models five or 10 years later. While they were good enough for early models, we had to continuously update the benchmarks, annotations and metrics to account for this, which of course could have been prevented if we were able to get better data, calibration and annotations in the first place.
What direct feedback—as opposed to citations—have you received in the decade since your paper appeared?
People have acknowledged KITTI in various formats. We have received great feedback across industry and academia on how KITTI has helped advance the field. Sometimes, I also received feedback that people were happy that their research efforts that have been going on for a long time are finally recognized, because self-driving is not a niche research area anymore, with specialized conferences and ignored by the majority of computer vision and machine learning researchers. Instead, it is a prominent citizen of the community, with various big workshops organized in conjunction with CVPR and other conferences, and a large number of papers published on this topic. We have also gotten a lot of feedback from follow-up datasets and benchmarks that have been inspired by KITTI.
How have others built on what you published? (And how have you yourself built on it?)
Apart from many follow-up datasets and benchmarks, a large number of papers have built upon our evaluations, to develop novel algorithms and models that are more robust and accurate across various perception tasks. We ourselves have continued research on those tasks, but also developed KITTI into KITTI-360, with larger sensor coverage and novel tasks, connection vision, robotics and graphics. For example, one task that is very popular today is novel view synthesis (popularized by NeRF) which we now also cover in KITTI-360 and which will enable new ways to train and validate self-driving systems to become more robust and achieve the necessary accuracy for human-level self-driving.
Could you name a paper (or other scholarly work) that has had the most, or at least a large, impact on you and your work?
KITTI developed as a side-project of my PhD thesis which was on urban traffic intersection understanding. For my PhD project, I had to record a lot of data, and spent about one year configuring the vehicle. I thought this effort should be more broadly useful and discussed the idea of extending the datasets into proper benchmarks with my advisors. At the time, I was also talking to David McAllester who was heading TTI-Chicago where I spent a lot of my time to better collaborate with my co-advisor Raquel Urtasun that also played a crucial role for establishing KITTI and making it popular and accessible. David was extremely excited by the premise that we could get some tasks like stereo, for which only very small-scale benchmarks (Middlebury benchmark) existed at the time, benchmarked at large scale, and that we could exploit the data to train larger machine learning models. We were also very inspired by the PASCAL-VOC challenges 1 that were seminal in establishing (at the time) large-scale benchmarks for object recognition with held-out test data and evaluation servers. In the end, we decided to make this effort a larger one, and we got lucky. We had something new that didn’t exist before and people picked up on it.