Statistics’ Crisis of Reproducibility
To cap the International Year of Statistics, last November 100 prominent statisticians attended an invitation-only event in London to grapple with the challenges and possible pathways that future presents. Earlier this month, Statistics and Science: A Report of the London Workshop on the Future of the Statistical Sciences, the product of that high-level meeting, was released by the six societies: the American Statistical Association, the Royal Statistical Society, the Bernoulli Society, the Institute of Mathematical Statistics, the International Biometric Society, and the International Statistical Institute.
In the following weeks, Social Science Space will excerpt portions of that report highlighting case studies on the current use of statistics and the challenges the discipline faces, such as the reproducibility crisis. (For a PDF of the full report, click here.)
Others in the series: Big Data: No Free Lunch for Protecting Privacy
***
One of the most controversial topics at the Future of Statistics workshop, after Big Data, was the problem of reproducibility in scientific research. While opinions vary as to how big the problem is, major science magazines and even the U.S. Congress have taken note.
In 2005, statistician John Ioannidis started the debate with a widely read and provocatively titled paper called “Why Most Published Research Findings Are False.” His conclusion was based on a simple mathematical argument, combined with an understanding of the way that statistical significance tests, specifically p-values, are typically used. (See “Reproducibility: Two Opposing Views” below)
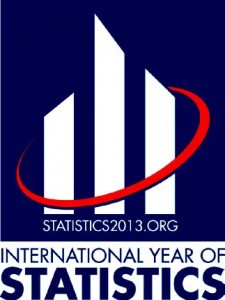
The London report examines a number of challenges and opportunities for the use of statistics, both within the milieu of Big Data –i.e. problems of scale, different kinds of data, privacy and confidentiality – and in the statistics world in general – i.e. reproducibility of scientific research, in controversial arenas like climate change, visualizing results and the rewards for professionals.
Though the details may be complex, a clear message emerges: A surprisingly large percent of claimed discoveries in the medical literature can be expected to be wrong on statistical grounds alone. Actual evidence based on the scientific merits suggests that the problem is even worse than advertised. Researchers from Amgen were able to replicate only six out of 53 supposedly classic results from cancer research. A team at Bayer HealthCare reported in Nature that when they tried to replicate 67 published studies, the published results were “completely in line with our in-house findings” only 14 times. These are all real failures of replication—not projected failures based on a statistical model. More recently, a network of nearly 100 researchers in social psychology attempted to replicate 27 studies. These were not obscure results; they were picked because of their large influence on the field. Nevertheless, 10 of the replication efforts failed completely and another five found smaller effects than the original studies.
These examples point to a larger systemic problem. Every scientific researcher faces pressure to publish articles, and those articles that report a positive new discovery have a great competitive advantage over articles with a negative result. Thus there is a strong selection bias in favor of positive results, even before a paper sees print.
Of course, the scientific method is supposed to weed out inaccurate results over time. Other researchers should try to perform the same experiment (this is called “replication”), and if they get different results, it should cast doubt on the original research. However, what should happen is not always what does happen. Replication is expensive and less prestigious than original research. Journals tend not to publish replication studies. Also, the original research paper may not contain enough information for another researcher to replicate it. The methodology may be described incompletely, or some data or computer programs might be missing.
In 2013, the journal Nature introduced new policies for authors of life-science articles, including an 18-point checklist designed to encourage researchers to be more open about experimental and statistical methods used in their papers. PLoS One, an open online journal, teamed with Science Exchange to launch a reproducibility initiative through which scientists can have their research validated (for a fee). “Reproducibility” has become a new catchword, with a subtle distinction from “replication.” In the era of Big Data and expensive science, it isn’t always possible to replicate an experiment. However, it is possible to post the data and the computer software used to analyze it online, so that others can verify the results.
The reproducibility problem goes far beyond statistics, of course, because it involves the entire reward structure of the scientific enterprise. Nevertheless, statistics is a very important ingredient in both the problem and the remedy. As Leek commented in a blog post, “The vast majority of data analysis is not performed by people properly trained to perform data analysis.” Along with all the other measures described above, scientists need more access to qualified statisticians—which means that more statisticians need to be trained. Also, the statistical training of subject matter experts (scientists who are not statisticians) needs to be improved. The stakes are high, because the more the public reads about irreproducible studies, the more they will lose their trust in science.
Reproducibility: Two Opposing Views
Here are the basic arguments that John Ioannidis used to claim that more than half of all scientific discoveries are false, and that Leah Jager and Jeffrey Leek used to rebut his claim.
Most Published Discoveries Are False. Suppose that scientists test 1,000 hypotheses to see if they are true or false. Most scientific hypotheses are expected to be novel, perhaps even surprising, so a priori one might expect 90 percent of them to be false. (This number is very much open to debate.) Of the 900 false hypotheses, conventional statistical analysis, using a p-value of 5 percent, will result in 45 being declared true. Thus one would expect 45 “false positives.” For the 100 true hypotheses, the probability of detecting a positive effect is called the “power” of the experiment. A typical target that medical researchers strive for is 80 percent. Thus, Ioannidis argued, 80 of the 100 true hypotheses will be declared true. These are “true positives.” Both the false and true positives are presented as “discoveries” in the scientific literature. Thus, 45 out of 125 (36 percent) published discoveries will actually be false. If the a priori likelihood of a hypothesis being true is smaller, or if the power is lower, then the false discovery rate could be more than 50 percent, hence the title of Ioannidis’ paper.
Most Published Discoveries Are True. Like Ioannidis’ paper, Jager and Leek’s estimate was not based on evaluating the actual scientific merit of any papers. However, it did go a step beyond Ioannidis in the sense that it was based on empirical data. They reasoned that for the false hypotheses, the p-value should simply be a random number between 0 and 1. On the other hand, for the true hypotheses, they argued that the p-values should follow a distribution skewed toward zero, called a beta-distribution. They collected all the p-values reported in every abstract published in five major medical journals over a 10-year span and computed which distribution best matched the p-values they found. The result was a mix of 14 percent false positives, 86 percent true positives. Hence, they concluded, about 86 percent of published discoveries in those five journals are true, with only 14 percent false.