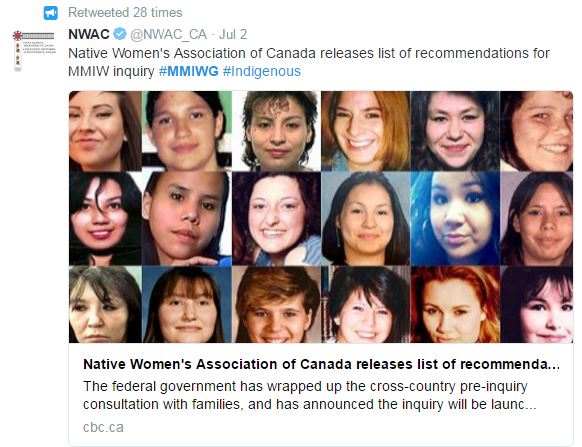
Use Qualitative Methods In Mining the Data Gold Rush
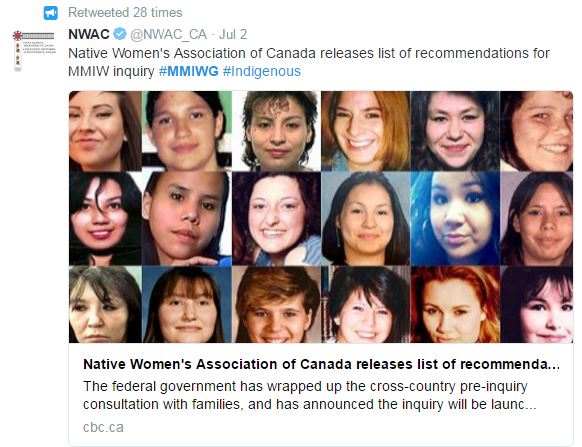
In her research Mylynn Felt combined qualitative methods with social media analytics to learn about grass-roots activism centered on Canada’s Murdered and Missing Indigenous Women (MMIW) campaign.
There’s a developing “data gold rush” for social science, and to address it a popular recent paper in the journal Big Data & Society calls for researchers to combine their traditional skills in qualitative methodology with the newly available social media data analytics.
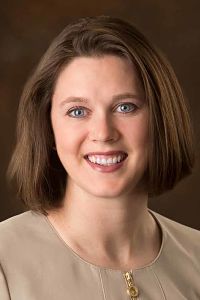
J. Mylynn Felt
As the pell-mell imagery of a gold rush suggests, there are riches to be found but much to be overlooked in the throes of information overload, writes author J. Mylynn Felt, a PhD candidate at the University of Calgary’s Department of Communication, Media & Film. “Just as light pollution in cities drowns out a view of the stars,” she writes, “too much instant data can flood the research focus, preventing a historical perspective. … It is vital that social scientists apply such critical analyses to utilize the vast potential of social media data for purposes of epistemology rather than the exploitation common in corporate and government Big Data analytics.”
The open-access paper initiated a sort of gold rush itself, as tweets and retweets of links to “Social media and the social sciences: How researchers employ Big Data analytics” hit numbers usually reserved for cat videos. Investigating what set social media savvy social scientists atwitter, we asked Felt to tell us more about mining at the intersection of social media analytics and qualitative approaches.
You open with the term “data gold rush” and I’m reminded that in past gold rushes the recurring motif is that the people who sold picks and shovels were quite successful in the long run. Do you feel something akin to that (even if the tools you’re discussing don’t benefit you)?
That is a good observation, and there are certainly many companies that have sprung up intending to fill such needs. It is also worth noting that Twitter has stopped offering its full data stream to data vendors. As a company, they seek to control that data commodity. However, it is still quite possible for researchers to gain free access to social media data. The scale of access is usually restricted by the social media platform in order to preserve data commodity value. Nevertheless, important research is still possible given the current free tools available.
Although we’re talking more about methods than output here, could you briefly describe what you were doing with Sisters in Spirit and what you learned?
My current research is part of a larger Social Science and Humanities Research Council-funded project designed to examine Canadian grass-roots civic engagement through social media. I am working on a comparative case study of three instances of Canadian collective action. The Murdered and Missing Indigenous Women (MMIW) campaign, which called for a national inquiry to the elevated rates of violence against Indigenous women of Canada, is one of the three cases I am examining. (Some of these preliminary findings will appear in the 2016 Social Media and Society conference proceedings.)
The MMIW campaign evolved over time and used many hashtags such as #MMIW, #MMAW, #MMIWG2S, and #AmINext, among others. This call for a national inquiry relied on rhizomatic organization and leadership. Individuals throughout the country activated local networks to organize embodied rallies and vigils such as the annual Sisters in Spirit March as well as internationally involved social media campaigns. Both the online and the offline events triggered mass media coverage and successfully generated enough general public attention to make MMIW a 2015 federal campaign issue.
You argue that data analytics should complement qualitative methods. Given tensions in the past between qualitative and quantitative partisans, how has this message been received?
There are often tensions between qualitative and quantitative perspectives. However, these are not irreconcilable. Much of the work social scientists do relies on qualitative methods. This allows for richer understanding of latent over manifest meaning in social media posts. There are limitations to any research method, whether qualitative or quantitative. Utilizing multiple methods helps to address the limitations of one method over another.
You have studied Twitter. What makes Twitter so appealing? Can you identify some of the challenges of using that particular social medium? Apart from discussions of specific application programming interfaces (API), do you feel your general observations are applicable across other social media?
Just as different platforms appeal to users for different purposes, so do they appeal differently to researchers. Twitter is a good place to find truly public information. Some research questions are better answered through the platforms that encourage more private community construction. Twitter is one platform in which posts, while initially visible only to those following a given account, are searchable by any users. Two of the top appeals of Twitter for a researcher are the public nature of communication and access to it.
All platforms create research challenges. One of the challenges with Twitter is in generating a data capture in a timely manner to collect all the relevant posts for a given topic. While each platform presents different possibilities and challenges, many of the observations I make in this article apply to most dominant social media platforms as well. Two of the three toolsets evaluated are designed to work with other platforms in addition to Twitter. The third is grouped with separate tools designed for other specific platforms. The call for social scientists to take a critical approach to big data analytics of social media relates to research of any platform.
How important is being able to visualize your findings and/or data?
Effective visualization is highly useful to telling the story a researcher sees in the data collected and interpreted. However, visualizations can be misleading or can appear more confusing to an audience. For example, word clouds are very popular and yet many researchers take issue with them. While they may demonstrate the frequency of words within a text, the order and coloring of clusters is often generated randomly. This can suggest relationships between terms that do not originate from the text but instead from the algorithm that created the word cloud.
When researchers choose to use the data visualizations automatically generated by various research tools, the choice should be purposeful. Quite often all of what can be shown is less useful that the few data visualization charts which effectively demonstrate a point of particular interest.
You studied voluntarily generated data. How do you navigate ethical, privacy and ‘institutional review board’-directed concerns with this ‘voluntary’ data?
There are many regulatory boards in place to ensure ethical use of voluntarily generated data for research. One of these groups is the university IRB. Another is the platform API conditions of use. In the case of this specific data set, the tweets related to the Sisters in Spirit Vigil were generated by a group seeking public attention for the sake of societal change. When the assumed purpose of public posting is to raise awareness, amplification of claims through academic research may help the cause.
Do you have any predictions about what we might see at the nexus of social science research and Big Data in the future?
I hope to see a growing increase in social science big data research. One way this might develop is through inter-departmental research partnerships. Social scientists ask different types of questions than many of the groups currently focused on big data. Certainly big data research will continue to grow in proportion with the level of engagement the general public places on social media. Social scientists continue to actively research the communicative, societal, and other aspects of social media use. I hope to see a growing blend of established qualitative techniques with newly emerging big data research methods. Whether such approaches develop and take hold will depend on many factors. One of these is the usefulness of early studies using this approach. Another is the willingness of established researchers to adopt new techniques and partner with others.