Good Replication Standards Start With the Data
How can we create reliable and replicable political science data? A recent article in the American Political Science Review focuses on text analysis and suggests ways to make these data sound and reproducible.
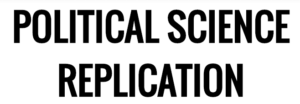
This post by Nicole Janz originally appeared at her blog, Political Science Replication, under the title, “Towards A More Comprehensive Replication Standard In Political Science: Reproducible Data Collection.” It is reposted here with her permission
Kenneth Benoit from the London School of Economics and his co-authors write in their article “Crowd-sourced Text Analysis: Reproducible and Agile Production of Political Data” that there is now a strong trend in political science, where journals ask authors to upload data and software codes for replication. However, there is still uncertainty on how to make the datacollection itself reproducible.
Most guidelines by journals require files so that replicators can reanalyze the given dataset and run or improve the software code. However, such an replication of an analysis “sets a far weaker standard than reproducibility of the data”. The authors propose that a “more comprehensive” replication standard should involve replication of the data collection and production itself.
This is an excellent point. I have discussed earlier on my blog that good practice for data collection entails to keep detailed logs about the sources and all procedures and decisions – including selection, merging, transforming and cleaning raw data. Without good practice in data collection, a replicator may be able to reproduce the analysis itself with an uploaded data set by the authors – but it may be impossible to follow the data creation process.
In their article, the authors show how reproducible data collection can be implemented for scholars engaged deriving data from of political texts. Most scholars in political science have come across and used such data in their training or research. I work a lot with human rights measurements such as the Political Terror Scale, which creates an index from 1-5 coding human rights violations by governments published in Amnesty International and U.S. State Department reports. I’ve also worked with data based on presidential speeches (although not quantitatively). Anytime you use such data without fully knowing the production process, you may not be able to replicate (or at least understand) the data collection, and therefore you may not want to trust these data.
The authors go on to show how they used expert and massive numbers of non-expert coders (via crowd sourcing) working on textual sources. In giving detailed criteria on how the data were analyzed, the authors demonstrate how a comprehensive replication standard can be implemented for such and any other political data collection process.
I highly recommend reading the full article:
KENNETH BENOIT, DREW CONWAY, BENJAMIN E. LAUDERDALE, MICHAEL LAVER and SLAVA MIKHAYLOV (2016). Crowd-sourced Text Analysis: Reproducible and Agile Production of Political Data. American Political Science Review, 110, pp 278-295. doi:10.1017/S0003055416000058.