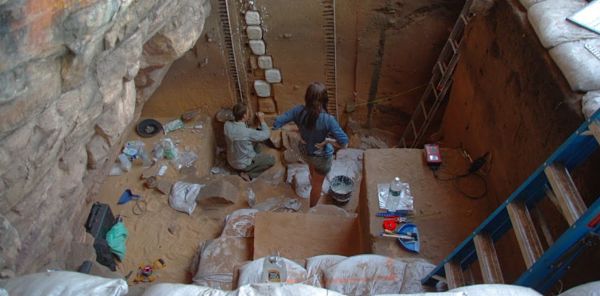
Addressing Reproducibility in Archaeology: Our Three-Pronged Approach
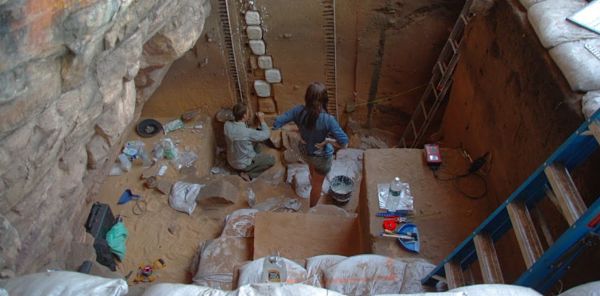
Step one is not being afraid to reexamine a site that’s been previously excavated.
(Photo: Dominic O’Brien/ Gundjeihmi Aboriginal Corporation/CC BY-ND)
“After a certain point, all that ridiculous information can make you wonder: is science bullshit? To which the answer is clearly no. But there is a lot of bullshit currently masquerading as science.”
A big part of this problem has to do with what’s been called a “reproducibility crisis” in science – many studies if run a second time don’t come up with the same results. Scientists are worried about this situation, and high-profile international research journals have raised the alarm, too, calling on researchers to put more effort into ensuring their results can be reproduced, rather than only striving for splashy, one-off outcomes.

This article by Ben Marwick and Zenobia Jacobs originally appeared at The Conversation, a Social Science Space partner site, under the title “Here’s the three-pronged approach we’re using in our own research to tackle the reproducibility issue”
For example, it informs what we know about how to stay healthy, how doctors should look after us when we’re sick, how best to educate our children and how to organize our communities. If study results are not reproducible, then we can’t trust them to give good advice on solving our everyday problems – and society-wide challenges. Reproducibility is not just a minor technicality for specialists; it’s a pressing issue that affects the role of modern science in society.
Once we’ve identified that reproducibility is a big problem, the question becomes: How do we tackle it? Part of the answer has to do with changing incentives for researchers. But there are plenty of things we in the research community can do right now in the course of our scientific work.
It might come as a surprise that archaeologists are at the forefront of finding ways to improve the situation. Our recent paper in Nature demonstrates a concrete three-pronged approach to improving the reproducibility of scientific findings.
Going back to where it all started
In our new publication we describe recent work at an archaeological site in northern Australia. The results of our excavations and laboratory analyses show that people arrived in Australia 65,000 years ago, substantially earlier than the previous consensus estimate of 47,000 years ago. This date has exciting implications for our understandings of human evolution.
A less obvious detail about this study is the care we’ve taken to make our results reproducible. Our reproducibility strategy had three parts: fieldwork, labwork and data analyses.

Ben Marwick and colleagues excavating at lowest reaches of
pit at Madjedbebe.(Photo: Dominic O’Brien. Gundjeihmi Aboriginal Corporation/, CC BY-ND)
Our first step toward reproducibility was our choice of what to investigate. Rather than striking out to someplace new, we reexcavated an archaeological site previously known to have very old artifacts.
The rockshelter site Madjedbebe in Australia’s Northern Territory had been excavated twice before. Famously, excavations there in 1989 indicated that people had arrived in Australia by about 50,000 years ago. But this age was not accepted by many archaeologists, who refused to accept anything older than 47,000 years ago.
This age was controversial from its first publication, and our goal in revisiting the site was to check if it was reliable or not. Could that controversial 50,000-years age be reproduced, or was it just a chance result that didn’t indicate the true time period for human habitation in Australia?
Like many scientists, archaeologists are generally less interested in returning to old discoveries, instead preferring to forge new paths in search of novel results. The problem with this is that it can lead to many unresolved questions, making it difficult to build a solid foundation of knowledge.
Double-check the lab tests
The second part of our reproducibility strategy was to verify that our laboratory analyses were reliable.
Our team used optically stimulated luminescence methods to date the sand grains near the ancient artifacts. This method is complex, and there are only a few places in the world that have the instruments and skills to date these kinds of samples.
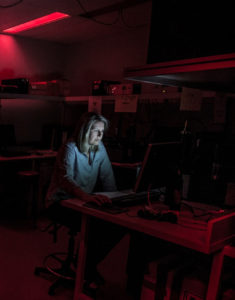
Zenobia Jacobs produced the new ages for the Madjebdebe site based on her work in the Luminescence Dating Laboratory at the University of Wollongong, Australia.(Photo: University of Wollongong/, CC BY-ND)
We first analyzed our samples in our laboratory at the University of Wollongong to find their ages. Then we sent blind duplicate samples to another laboratory at the University of Adelaide to analyze, without telling that lab our results. With both sets of analyses in hand, we compared them; it turned out in this case that they got the same ages as we did for the same samples.
This kind of verification is not a common practice in archaeology, but because this site was already controversial, we wanted to make sure the ages we obtained were reproducible.
While this extra work involved some additional cost and time, it’s vital to proving that our dates give the true ages of the sediments surrounding the artifacts. This verification shows that our lab results are not due to chance, or the unique conditions of our laboratory. Other archaeologists, and the public, can be more confident in our findings because we’ve taken these extra steps. This external checking should be standard practice in any science where controversial findings are at stake.
Don’t let the computer be a black box
After we completed the excavation and lab analyses, we analyzed the data on our computers. This stage of our research was very similar to what scientists in many other fields do. We loaded the raw data into our computers to visualize it with plots and test hypotheses with statistical methods.
However, while many researchers do this work by pointing and clicking using off-the-shelf software, we tried as much as possible to write scripts in the R programming language.
Pointing and clicking generally leaves no traces of important decisions made during data analysis. Mouse-driven analyses leave the researcher with a final result, but none of the steps to get that result is saved. This makes it difficult to retrace the steps of an analysis, and check the assumptions made by the researcher.
On the other hand, our scripts contain a record of all our data analysis steps and decisions. They’re like an exact recipe to generate our results. Other researchers not using scripts for their data analysis don’t have these recipes, so their results are much harder to reproduce.
Another advantage of our choice to use scripts is that we can share them with the scientific community and the public. We follow standard practices by making our script files and main data files freely available online so anyone can inspect the details of our analysis, or explore new ideas using our data.
It’s easy to understand why many researchers prefer point-and-click over writing scripts for their data analysis. Often that’s what they were taught as students. It’s hard work and time-consuming to learn new analysis tools among the pressures of teaching, applying for grants, doing fieldwork and writing publications. Despite these challenges, there is an accelerating shift away from point-and-click toward scripted analyses in many areas of science.
Combating irreproducibility one step at a time
Our recent paper is part of a new movement emerging in many disciplines to improve the reproducibility of science. Examples of recent papers that have made a commitment to reproducibility similar to ours have come from epidemiology, oceanography and neuroscience.
We hope our example will inspire other scientists to be strategic about improving the reproducibility of their research. Some of these steps can be difficult for researchers: It means learning how to use unfamiliar software, and publicly sharing more of their data and methods than they’re accustomed to. But they’re important for generating reliable results – and for maintaining public confidence in scientific knowledge.