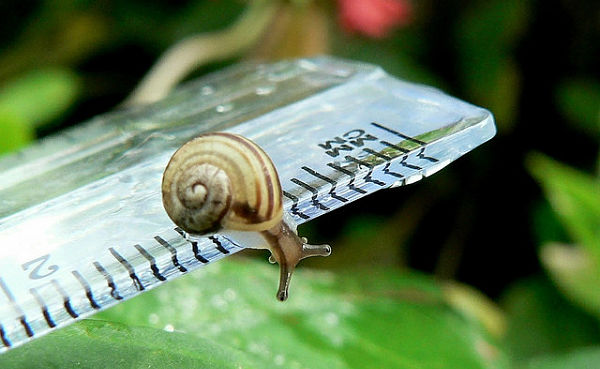
Academe Just Doesn’t Talk Enough about Research Metrics
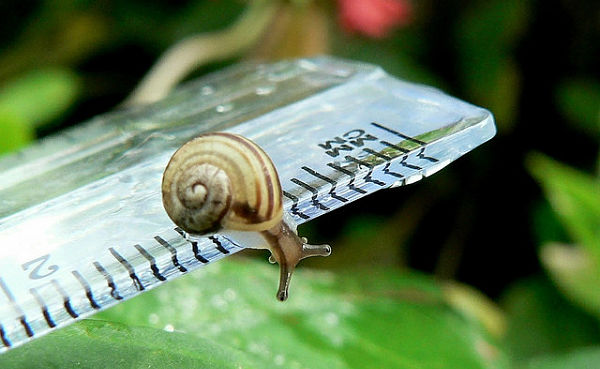
Two years ago, I conducted a study about the implications of evaluative metrics on research practices. I asked participants – with representation from across all career stages, from postdocs to professors – what they understood about metrics, and how metrics would and could affect their research practices. In the interviews, time was a common topic. Participants often mentioned that “time is short”, that there was “not enough time”, or that ”I don’t have time for…” Although we cannot establish whether there is a correlation between the use of evaluative metrics and time, the frequent mention of time pressure made me wonder about an interesting finding of the study — the distinction between discussion of evaluative metrics in principle and in practice.
In principle, most participants in the study were concerned about the limitations of evaluative metrics and the extent of their use. They talked about how metrics should not be used, how they are not comparable, how they encourage gaming behaviour, and so on.
In practice, they track their own metrics, and use metrics to evaluate their own productivity and the quality of their work. It is also seemingly unavoidable to use metrics to evaluate applications for academic positions and research grants.
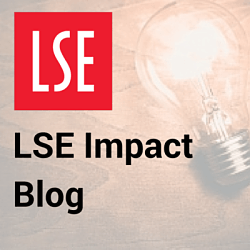
So, why is there such a discrepancy between their principle and practices pertaining to evaluative metrics? Their active uses in everyday academic and research activities seem to indicate that academics have accepted metrics as standards of evaluation, and that they are “thinking with indicators” as Ruth Müller and Sarah de Rijcke suggest. In other words, participants are following rules that are implicit and embedded in everyday academic life. Yet, when they are asked to reflect on the use of metrics, they articulate their limitations and inappropriateness. That led me to develop two related concepts: evaluation complacency and evaluation inertia.
Evaluation complacency — when one is complacent about the achievements measured by evaluative metrics, not feeling the need to reflect on the limitations and shortcomings of metrics. Implicit in evaluative metrics is an acceptance of metrics as standards, as objective, fair measures of research quality, productivity, and performance. Such an acceptance can be a result of complacency in a system where the rich get richer, and hence reinforce the system.
Evaluation inertia — when there is no tendency to reflect, critique, or change the existing standards of research evaluation, including the use of metrics, because, for example, the system encourages the chasing of metrics as a goal. As the competition intensifies (e.g. one needs a higher number of publications to secure an academic position), there is no time or headspace to reflect and critique existing standards and practices — which leads to the lack of discourse about metrics, among other things.
Why is discourse important? In The Theory of Communicative Action, Jürgen Habermas describes what is called an ideal speech situation, one in which every agent has equal opportunity and right to express and negotiate meanings — of language, of action. Habermas asserts that an open and public discourse is the basis for democracy.
What, then, of metrics? How should they be constructed? How should they be used?
This blog post is based on a paper the author presented at the fourth Accelerated Academy conference, Academic Timescapes: Perspectives, Reflections, Responsibilities, held May 24-25, 2018, in Prague, and funded by The Czech Science Foundation (grant no. 16-18371Y), Czech Academy of Sciences (Strategie AV21), Portuguese Science Foundation, and CECS – University of Minho.
How can we answer these questions? Ideally, as in Habermas’ ideal speech situation, these questions should be negotiated in public discourse, featuring agents including academics, researchers, university administrators and management, funding agencies, publishers, commercial database and index providers, the government, and even the general public. The discourse about metrics, however, seems to be largely limited to specialists such as bibliometricians and some science and technology studies scholars. What we haven’t heard too much is the discourse among those who contribute to and use metrics heavily, those who are directly affected by metrics when looking for positions and applying for grants or promotions. That is, academics themselves, particularly those in junior positions.
Why?
“I don’t have time,” they say.
However, if academics do not believe in the objectivity, appropriateness, or legitimacy of metrics in evaluation, why don’t they say something? Why do they continue to engage in gaming behavior? To what extent can we describe evaluation complacency and evaluation inertia as part of our everyday academic lives?
“Time is short” is perhaps both a symptom and a cause of the lack of discourse around metrics. When the use of metrics in research evaluation prompts us to produce more and faster, we feel that we don’t have enough time and consequently don’t spare time to think about whether the use of metrics is right or wrong, good or bad. The more metrics become institutionalised and ritualised, the more power and control they will exert in academic life, to the extent that we experience evaluation complacency and/or evaluation inertia.
I’d like to suggest that we bring the discourse about metrics into our everyday academic lives. The understanding of metrics should not be limited to specialists. There is an urgent need for everyone who uses and is affected by these metrics to be fully informed and to engage in conversations in an open and public discourse. A possible first step is to introduce evaluative metrics as part of doctoral studies. Recent publications by Sugimoto and Larivière and Rousseau, Egghe, and Guns, as well as the Metrics Toolkit are tailored for researchers and others who are not specialised in bibliometrics/scientometrics, for example. Rather than accepting the use of evaluative metrics as rules and standards, academics can become more reflective and critical in everyday use, and more deliberate about the importance of evaluative metrics as collaborators, peer reviewers, and administrators.