How Could Google Scholar (and the Citation System) Be Improved?
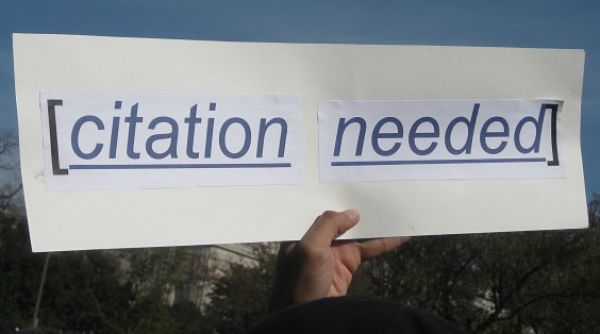
In this third and final article on Google Scholar, I’ll explore how the citation system, and Google Scholar in particular, might be improved. One key question is whether the current research citation system, including Google Scholar, treats all researchers fairly e.g. female, non-English-speaking, Global South, or early-career researchers?
On the first three, the answer is probably no, but perhaps it could be argued they are such systemic issues it’s not Scholar’s problem to fix alone. Women are penalized for career breaks in nearly all industries (I’d love to hear views on what could be done about this with research impact). On languages and non-Western countries, Scholar could point out the 11 other languages it’s added to its Metrics feature (as well as the Google Translate service), it’s huge scope, and the way it makes research online discoverable.
When it comes to early-career researchers, it’s ironic that although the small nine-person Scholar team is focused on ensuring early-career researcher and newly-published content can be found easily, it’s still the case that a longer research track-record can create what is called a “denser graph”, with stronger signals for web crawlers – thus putting early-career researchers and new content at a disadvantage. The combined ranking algorithm emphasises citation counts and title words, strengthening the Matthew effect of accumulated advantage so that the (academically) rich get richer, while the poor get poorer. The current iteration of the “Metrics” function doesn’t include publications with fewer than 100 articles from 2013-17.
It’s not clear what can be done about this as Scholar won’t share how its algorithms work. But the “standing on the shoulders of giants” motto starts to have a double-meaning, with Lilliputian new researchers and publications facing the daunting task of scaling their better-established and relatively gigantic peers.
Hierarchies
Another question is whether all disciplines and research methods are treated equally by Scholar (and whether they should be)? The h-index can be systematically different for each discipline e.g. higher in STEM than social science, or even within subfields of the same discipline. Also, citation lag-times can vary by discipline e.g. be faster in life sciences than Geography.
And what about those disciplines more reliant on summaries/book chapters than pure research? Or those disciplines that are more ‘established’, ‘higher-paradigm’, or just bigger – with more researchers, publications and documents? Is it just the case that even novel fields should integrate with existing research structures and models e.g. digital humanities scholars with experimental resources or computer scientists producing ground-breaking new code, which are hard to index and don’t fit with existing systems, need a standard way to showcase content and so should “play the game” e.g. journals. Academic Search Engine Optimization (ASEO) practices can also help as web crawlers need standardized structures and metadata.
In Google Scholar the best performing citations and journals are the most transparent – with machine-readable and up-to-date metadata e.g. full xml, chapter-level metadata, full references in books, an appropriate reference style structured with all the information and not broken-up or buried in slow-to-crawl books (often reliant on Google Books). Although many of those involved in academic content use ASEO for crawling/indexing by Google Scholar and other services, more organisations are encouraged to engage directly with Google Scholar e.g. to make sure they’re using industry-supported metadata formats. However, this again tends to favour bigger, more established content producers – giants if you will.
Beyond the numbers
So how might the current citation metric system, including Google Scholar, be improved? One approach is to add additional – qualitative and/or quantitative – information, for instance to author profiles in Scholar e.g. author mentions in qualitative REF impact case studies (25 percent of REF 2021 funding), AltMetric’s fifteen attention measures, or Anne-Wil Harzing’s individual annualized h-index? Could researchers play a bigger role in validating others’ research, akin to the recommendation and skills functions in LinkedIn, or would these be open to abuse?
Although Scholar has considered how to make publicly-funded research more identifiable or available, for funders to see, that has proved a hard problem to fix. The small team have a long list of things to work on and have focused on helping researchers rapidly discover the right content, to make it relevant to them, whatever stage of their scholarly career they’re at.
It’s clear that Google Scholar is good at what it sets out to do, meeting many of the needs of its target users. However, it can also reflect those users’ (and as a result the wider system’s) biases, emphasising some of the subjective and hierarchical aspects of scholars, and well … people. What’s not clear is where Scholar’s responsibilities lie in creating a system that does more to encourage the best in people rather than prey on their faults. We’re in an era where those companies able to get ahead of that curve will have a profound lasting advantage, and vice versa.
I hope you’ve enjoyed this three-parter on how the current citation system – and Google Scholar in particular – judges research impact, constructive feedback is always welcome.