2018 SAGE Concept Grant Winners Interview- Digital DNA Toolbox
Following the launch of the SAGE Ocean initiative in February 2018, the inaugural winners of the SAGE Concept Grant program were announced in March of the same year. As we build up to this year’s winner announcement we’ve caught up with the three winners from 2018 to see what they’ve been up to and how the seed funding has helped in the development of their tools.
In this post, we spoke with the Digital DNA Toolbox (DDNA) winners, Stefano Cresci and Maurizio Tesconi about their initial idea, the challengers they faced along the way and the future of tools for social science research.
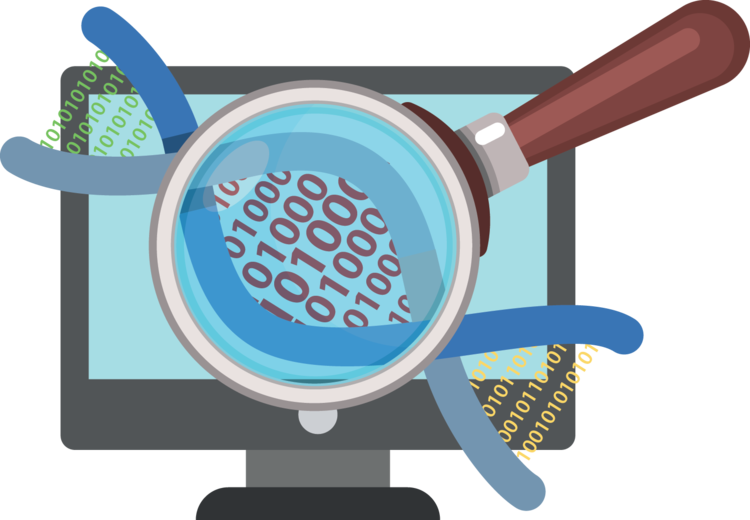
WHAT IS DDNA AND HOW DID YOU START OR COME UP WITH THE IDEA?
The Digital DNA (DDNA) Toolbox is a set of methods developed to support scientists in making sense of online data. In particular, it is designed for assessing the reliability of accounts and content (e.g., detecting fake and bot accounts) in online social networks (OSNs).
The methods provided by DDNA analyze similarities in online behaviors. When we first thought about studying online behaviors, we modeled them as sequences of actions. Then, we thought that the sequence of actions of an account could be represented with a string of characters, similarly to a string of biological DNA. Based on this idea, we then applied string mining and bioinformatics algorithms to the study of our “digital DNA” strings, with surprisingly good results!
Over the past two years, what were your main challenges and how did you overcome them?
One of the main challenges in detecting fake information is that online fake content, be it a piece of news or a fake account, are so accurately engineered as to often appear credible, if not investigated thoroughly. In other words, it is becoming increasingly difficult to tell apart single fake news and accounts from credible ones.
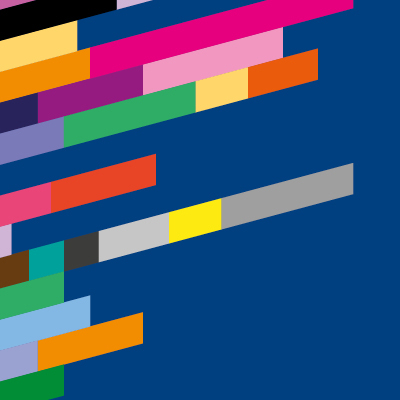
Because of this challenge, we wanted to fight the battle against fakes in a more favorable scenario. Thus, we switched from analyzing single fake items to analyzing groups of items. We followed the intuition that an exceptional amount of similarity between different items could serve as a red flag for automated/forged content. By analyzing groups we thus had more information for our analysis, which ultimately yielded positive results.
Why does your package resonate so well with social science researchers?
Many researchers make use of social media and OSN data for their studies. However, the tools for assessing the credibility of online data are still few and far between. Moreover, they are usually designed by technicians for technicians and thus they remain confined within the computer science community. Thanks to the funding from the SAGE Concept Grant, we had the opportunity to release our methods in the convenient form of both a Python and R package. We are confident that social science researchers will find it easy and useful to exploit the cutting-edge algorithms and techniques contained in the DDNA package.
Do you have any interesting examples or case studies to share?
Since we started experimenting with DDNA, we found many cases of online discussions tampered by bots and filled with fake content. One such case was the UK’s 2016 EU membership referendum. By applying our techniques to a small subset of tweets containing the #Brexit hashtag, we found several hundreds of bot accounts that were frantically tweeting in the weeks before the vote. Interestingly, all such accounts completely stopped tweeting after the Brexit vote.
In another recent study we used DDNA to uncover large botnets that try to artificially inflate the popularity of low-value stocks traded in US financial markets. The bots create a large number of fictitious tweets, where they mention low-value stocks with some high-value ones (e.g., Google, Apple, etc.). In this way, they give the impression of large discussions and widespread interest in the low-value stocks, in an effort to fool automatic trading algorithms and unaware investors.
What sets you apart from other tools and services in this space?
Unfortunately, there aren’t many services and tools for assessing the veracity and credibility of online data. To this regard, the DDNA is among the first available tools. The only similar service is Botometer, which is a public bot detection service. With respect to Botometer, the techniques included in the DDNA package obtained better fake detection results.
How did you hear about the sage concept grants, what made you apply and how did the funding help you bring your idea closer to a fully operational tool or package that researchers can use?
A fellow computer science researcher stumbled upon the SAGE Concept Grants and thought we might be interested, so he let us know. And actually, he was totally right since we found this initiative very useful. At that time, we lacked the effort to re-engineer our detection techniques into something that could be used by experts and non-experts alike. The funding received from SAGE helped us re-engineer our prototypical code and allowed us to develop 2 packages (i.e., a Python and an R package).
What’s your take on the future of tools for social science researchers?
Many of the critical challenges that we are facing today require an interdisciplinary approach. Empowering social and political scientists with the best algorithms and techniques developed by computer scientists allows us to be better prepared to face these challenges.. However, commendable initiatives such as the SAGE Concept Grants are still a rarity. For the future, we hope that many more similar initiatives will take place, in an effort that will hopefully contribute to reducing the gap between the pioneering tools developed by computer scientists, and the many other researchers that make use of online data.
Where can researchers find your tool and can they use it already?
A first preliminary version of our Python package is already publicly available for download and experimentation! We are now also nearing the release of the first version of the R package. We welcome all interested researchers to experiment with DDNA and to let us know of possible ways to improve it.
WHAT ARE YOUR NEXT STEPS AND HOW CAN OUR READERS GET INVOLVED?
We are always trying to improve the techniques at the core of DDNA. In particular, we are now working towards making DDNA more scalable, in order to allow more convenient large-scale analyses. Interested readers can keep an eye on our publications, as we constantly use DDNA for our research. Finally, we welcome all suggestions so don’t hesitate to drop us an email or to tweet about DDNA!
DDNA is being developed by Stefano Cresci and Maurizio Tesconi from the Institute for Informatics and Telematics, Italian National Research Council.