Measuring and Modeling the Unobservable
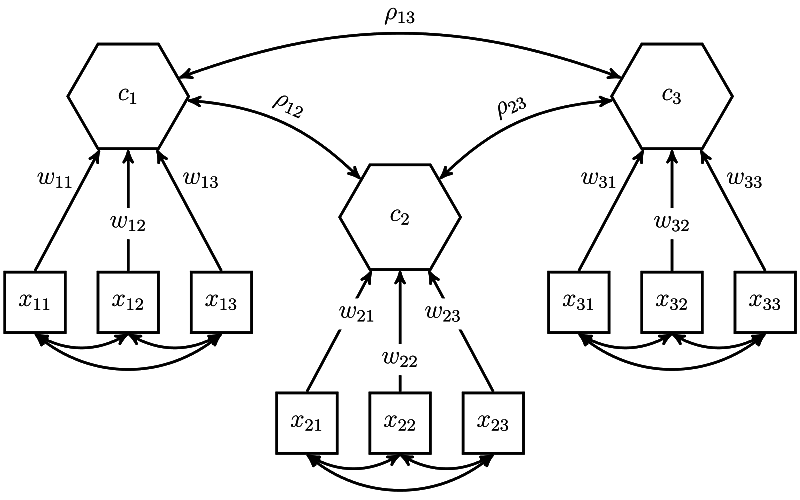
Today we welcome Professor Doctor Marko Sarstedt, chair of marketing in the faculty of economics and management at Otto-von-Guericke-University Magdeburg, discusses “Factors versus Composites: Guidelines for Choosing the Right Structural Equation Modeling Method,” an article Joseph F. Hair, Jr. and he wrote for Project Management Journal. The abstract appears below.
Structural equation modeling (SEM) is a widely applied and useful tool for project management scholars. In this Thoughtlet* article, we critically reflect on the measurement philosophy underlying the two streams of SEM and their adequacy for estimating relationships among concepts commonly encountered in the field (e.g., team performance). We also discuss considerations to ponder when making the choice between the two types of SEM as well as between SEM and regression analysis.
* Thoughtlets are short articles that propose cross-fertilization of domains or promote new directions that might seed ideas for stimulating project management research.
Many areas of research interest in project management and business research involve drawing conclusions about unobserved concepts such as client satisfaction, organizational culture, and team performance. In their effort to map these unobserved concepts in their statistical models, researchers often have a reflex-like preference for using common factors and common factor-based structural equation modeling (SEM), which has been viewed as “accounting for measurement error.” In contrast, using composites of observed variables to represent these concepts in tandem with composite-based SEM methods is often viewed as suspicious of not being able to (adequately) account for measurement error. Many scholars have always been concerned about this notion, not so much because of the measurement error argument – which, by the way, has been debunked – but rather because of the assumption that common factors are THE gold standard in measurement.
There is no doubt that factor theory with its origins in the early works of Charles Spearman offers a comprehensive framework for facilitating sound measurement. But researchers have also been quick to overlook the limitations of common factor theory and the advantages of using composites in statistical models. This thinking appears to be changing, however, as several articles in prominent outlets such as Journal of the Academy of Marketing Science, Journal of Business Research, and Psychological Methods have made a convincing case for composites. At the same time, recent research shows that a phenomenon known as factor indeterminacy, which is inherent in common factor-based methods, can have adverse consequences for the validity of common factor-based SEM. More precisely, factor indeterminacy produces a band of uncertainty in the relationship between a measured common factor and the concept it represents, which implies that the factor is not in fact as accurate a representation of the concept a previously thought.
We believe the implications arising from this recent research have the potential to change the debate regarding the relative merits of the several SEM methods, which started in the 1980s. Most research in this field has focused on aspects such as small sample size, parameter bias, and statistical power, often overlooking the fact that the methods are divergent in how they conceptualize latent variables; that is, by means of common factors or composites. This emerging thinking has broad implications for drawing conclusions about the relative advantages of SEM methods, which we discuss in our Thoughtlet article “Factors vs. composites: Guidelines for choosing the right structural equation modeling method.” Our argument that the latest developments pave the way for methods such as generalized structural component analysis and partial least squares SEM is certainly provocative, but we hope that it triggers some follow-up discussions and research delving more deeply into the nature of composite modeling and its philosophical underpinnings.