What Constitutes Gender Bias In Academia?
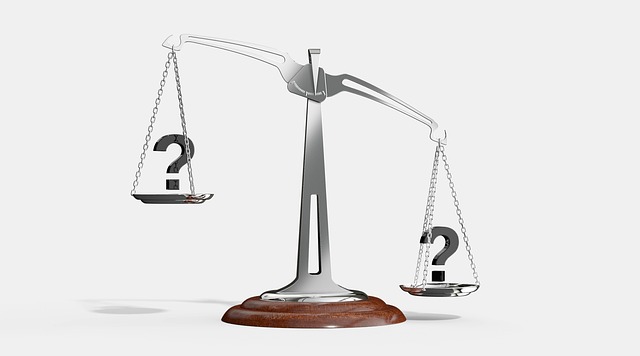
Equality, diversity and inclusion are pressing issues for research and higher education institutions. To ensure that research does not inadvertently harm underrepresented groups, Nature recently introduced new guidelines for studies engaging with questions of race, ethnicity or gender. Nature calls on researchers to reflect on these concepts and to elaborate on how they are defined and measured. For example, if gender is included in a study, is it self-reported or is it inferred using machine-learning algorithms? In addition, Nature asks researchers to explain how they controlled for confounding variables. Why is controlling for confounding variables so important, and why is it so challenging to do this correctly?
Let us consider the case of gender bias in academia. Researchers regularly observe gender differences in favor of men in various parts of academia, such as fewer women in senior academic positions, fewer publications, lower citations rates and lower funding of women. However, researchers also observe differences in favor of women, such as more women being elected in NAS, more favorable peer review and higher funding rates of women. Drawing conclusions about gender biases based on these differences is highly complex, not in the least because it often remains unclear what is meant by “bias.” This raises intricate questions about confounding variables and how to control for them.
What do we mean by bias?
In our recent work, we propose to define bias as an unjustified direct causal effect. This is a fairly technical definition, based on ideas from the field of causal inference. The essence of this definition is that there is a bias in the treatment of people with a particular characteristic, such as a particular gender, if they are unjustifiably treated differently because of that characteristic. For example, we say there is a gender bias in funding if the exact same grant proposal, with the same CV and the same track record, would be funded if the proposal were written by a man instead of a woman (or the other way around). If the exact same proposal is evaluated the same, regardless of the gender of the applicant, there is no gender bias.
Gender bias may lead to other problems down the line, as illustrated in the figure below. Suppose there is gender bias in the hiring of new research staff at a prestigious research institution. Men are more likely to be hired than women. Suppose also that researchers at prestigious institutions are more likely to receive external funding than equally qualified researchers at other institutions. This will then result in fewer women receiving funding. Even though there is no gender bias in funding, the gender bias in hiring at the prestigious institution has a negative indirect effect on the chances of women to receive funding. To distinguish this indirect effect from a direct effect, we call the indirect effect a gender disparity in funding, not a gender bias.
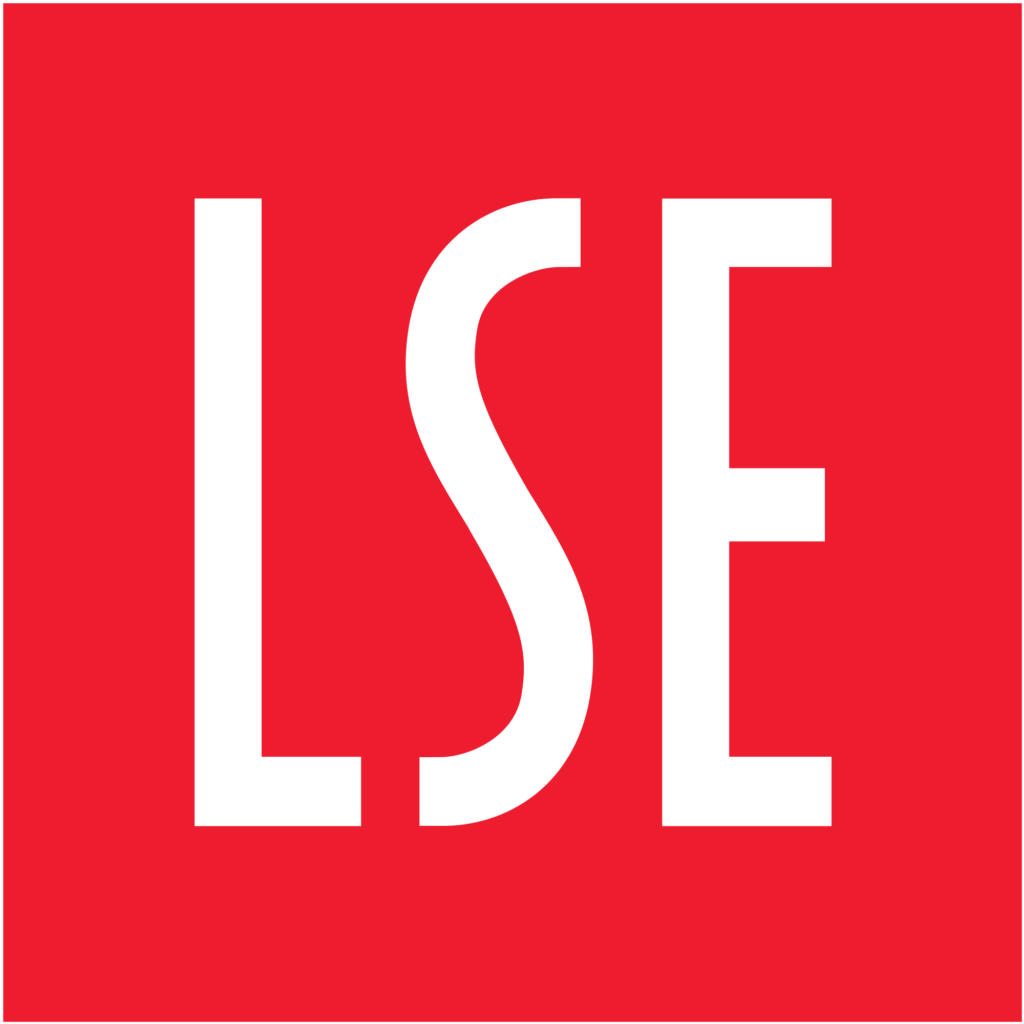
Difference does not imply bias
Just as correlation does not imply causation, our definition of bias highlights that gender differences do not imply gender biases. This is why controlling for confounding variables is necessary to make correct inferences about gender biases. At the same time, controlling for the wrong variables leads to incorrect inferences.
Consider again the above-mentioned case of gender bias in hiring, in which women have to pass a higher bar than men to be hired at a prestigious institution. A consequence of this gender bias is that women hired at the prestigious institution will on average be better qualified than men. As a result, relatively more women than men will receive funding at this institution, as shown in the above figure. This gender difference in funding does not imply a gender bias against men in the funding competition. It is simply a consequence of the gender bias against women in hiring.
Offering sound policy advice
The distinction between gender differences, gender disparities and gender biases is crucial to make sure researchers offer sound advice to policy makers. For instance, suppose researchers mistakenly conclude there is a gender bias in funding in the example presented in the above figure. When they inform policymakers about their conclusions, the policymakers may attempt to improve the funding process. This will be ineffective, since there is no gender bias in funding. There is a gender disparity in funding driven by a gender bias in hiring at the prestigious institution. Instead of trying to improve the funding process, policymakers should therefore focus on improving the hiring process at this institution. Addressing gender bias in hiring is not only important in itself, it will also address the gender disparity in funding.
However, a deeper analysis may reveal there actually is no gender bias in hiring at the prestigious institution. Instead, the gender difference in hiring may emerge elsewhere. For instance, suppose that on average women spend more time on childcare than men, and as a result, women publish less than men, who spend their evenings writing articles. If having more publications makes it more likely to be hired, we will see more men being hired. This is not a gender bias in hiring, but the result of a difference between how men and women spend time between childcare and scientific publishing.
Now suppose policymakers impose a hiring quota for women. How will this affect who gets hired? Imposing a quota may actually have an effect that runs counter to what is intended. If hiring decisions are still largely based on publications, imposing a quota will increase the chances for women to be hired, but the women benefiting from this will be particularly those who prioritize publishing over childcare. The quota will decrease the chances for men, in particular for those who prioritize childcare over publishing. While the gender balance will improve, the quota will only reinforce the idea that spending time on childcare hurts career chances, especially so for men.
Towards a robust evidence base
Effective policy to improve gender equality and gender diversity requires careful reflection on the causes behind the gendered patterns we see. Observational studies inevitably run into the challenge of causal inference. Experimental studies may alleviate some of these difficulties, but they tend to be difficult to carry out in real-world settings. Qualitative studies may address some of the intricacies around causality, but run into other limitations. Triangulating evidence from these different approaches seems our best bet to develop a robust understanding of gender biases.
Our proposed terminology offers a way to carefully distinguish between biases and disparities, which represent direct and indirect causal effects, and differences, which are not necessarily of a causal nature. This terminology aims to facilitate a clearer discussion about issues related to gender, race and ethnicity. We hope that researchers will take up our terminology, and the underlying causal perspective, to implement Nature’s new guideline on controlling for confounding variables.