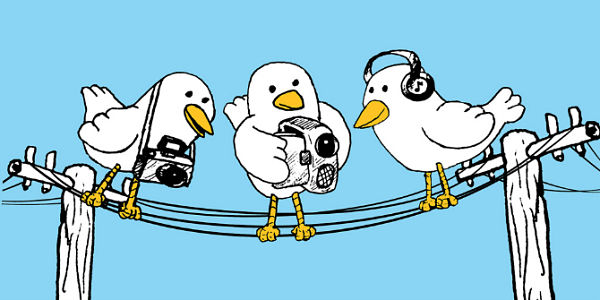
Little Blue Birds of a (Disciplinary) Feather Flock Together
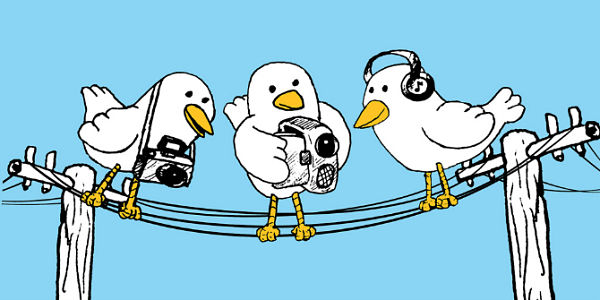
(Image: “Multimedia Birds of a Feather” by James Nash Flickr/CC BY-SA 2.0
Is science trapped in the ivory tower? Are scientists locked in their silos? How scientific knowledge reaches diverse groups beyond its own scientific community is an enduring question, one that is now positioned in a new context because of the rapid adoption of social media. As social media replaces traditional communication channels, it provides a completely new medium via which diverse groups can directly talk to each other, where a single message can potentially reach millions of people within an hour, and provides scientists with revolutionary ways to make detailed quantitative observations on communication at a global scale. As a result, many attempts, often collectively called “altmetrics,” have been made to capture the broad scientific impact beyond academia using social media data. The metrics have been heralded to measure societal impact of research and to complement traditional citation measures for research evaluation.
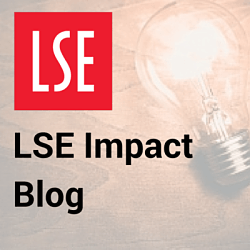
This article by Qing Ke, Yong-Yeol Ahn and Cassidy R. Sugimoto originally appeared on the LSE Impact of Social Sciences blog as “Scientific birds of a feather flock together: science communication on social media rarely happens across or beyond disciplinary boundaries” and is reposted under the Creative Commons license (CC BY 3.0).
Progress on this topic has been hampered by a lack of information about the producers of scientific discourse on social media and their networks. For instance, what if all social media sharing of a research paper were by automated bots? Or if all attention came from the author, journal, and publisher of the paper? Are these indicative of “broader impact”? Furthermore, perhaps all the attention is from scientists, but within the same domain: if all tweets about a paper on underwater basket weaving were from a tight clique of underwater basket weaving researchers, is this representative of the broader impact of science?
It is with these questions of broader impact that we began our research. We were curious to know how much scientific discourse is happening across and beyond scientific communities on social media. To do this, we started with a seemingly simple question: can we generate a list of scientists on social media? This is an inversion from previous research which began with a list of scientists (e.g. from bibliometric data) and then tried to find these individuals on social media. This previous approach led to a host of biases, such as prioritizing those who were successful in other metrics (e.g. production and citation), issues with data accessibility, as well as technological complications (e.g. author name disambiguation). Our approach was anchored within the platform, leveraging the wisdom of the crowds in terms of Twitter lists. Our underlying rationale was that we can safely consider a user as a scientist if (1) other users consider this person a scientist and (2) the person identifies as a scientist in their profile.
Read more
This blog post is based on the authors’ article, “A systematic identification and analysis of scientists on Twitter,” published in PLoS ONE
We were faced with the Herculean task of creating a list of scientific titles. We took a liberal approach, merging the classification from the US Bureau of Labor’s Standard Occupational Classification and scientific occupations in Wikipedia to prepare a list of “seed” scientists. Our final list of titles reveals interesting patterns about self-identification and specialisation of scientists on Twitter. First, our list identifies more practitioner-oriented disciplines than other disciplinary classifications. Secondly, our list demonstrated the role of specialisation in self-identification on Twitter: e.g. historians, by and large, identified as historians; chemists and biologists, on the other hand, identified with a large variety of specialised titles. This differentiation creates problems for identifying disciplinary populations of parallel scale; though this is not an uncommon problem for scientometric research.
Our seed list repeatedly matched the titles with Twitter list names and added newly discovered scientists. This process resolved in a sample of 45,867 scientists. Our method has been critiqued on the basis that certain disciplines may be underrepresented, using as evidence the comparatively large number of followers of scientific societies and journals. However, as has been demonstrated, a substantial proportion of scientific tweets are generated from bots, and organisational Twitter handles are likely to draw a large number of both bots and organisational followers. We therefore prioritized precision over recall – our objective was to create a replicable and systematic (rather than anecdotal) approach to identifying individuals who were likely to be scientists.
Given a sample of scientists, several questions can be answered that yield additional insight into the composition and behavior of the scientific community on the platform. What are the demographics of scientists on Twitter? What is the distribution across scientific disciplines? How is this population biased compared with the actual population? We automatically inferred gender of the scientists using first names and US Census data. The resulting data suggested that female scientists are overrepresented on Twitter relative to their representation in the scientific workforce. This may suggest greater avenues for participation in scientific discourse for women on this platform, though it would be necessary to control for age and other variables to fully understand this phenomenon. In terms of discipline, social and computer and information scientists are overrepresented, whereas life, physical, and mathematical scientists are underrepresented, compared with the US workforce. As has been suggested, it may be useful to replicate this method using other occupational classifications, to examine whether the results hold.
Some approaches to identifying scientists rely on the content of tweets. Therefore, using our verified list of scientists, we wanted to know the degree to which they tweeted about science and what other sources frequented their tweets. It turns out that scientists are people, too: the vast majority of what they share is the same as the general population. Social sites such as Instagram, Facebook, and YouTube, and major news sites such as The Guardian, The New York Times, and The Huffington Post are common sources. At the same time, it is clear that they share content relevant to their disciplines: the arXiv preprint server and the American Physical Society website are popular among physicists, the Association for Computing Machinery website among computer scientists, and the London School of Economics and Political Science blogs among social scientists.
This leads us to the final and perhaps most important question of our analysis. Do scientists form strong cliques based on their disciplines? We looked at how the scientists followed, retweeted, and mentioned each other. Our results showed high degrees of disciplinary assortativity—that is, scientific birds of a feather do indeed flock together. This has critical implications for the interpretation of social media metrics as metrics of broader or social impact. Our results suggest that social media does not broaden scientific communication, but rather replicates and perpetuates pre-established disciplinary boundaries. “Alt”-metrics may not be so alternative after all.