No More Tradeoffs: The Era of Big Data Analysis Has Come
For centuries, being a scientist has meant learning to live with limited data. People only share so much on a survey form. Experiments don’t account for all the conditions of real world situations. Field research and interviews can only be generalized so far. Network analyses don’t tell us everything we want to know about the ties among people. And text/content/document analysis methods allow us to dive deep into a small set of documents, or they give us a shallow understanding of a larger archive. Never both. So far, the truly great scientists have had to apply many of these approaches to help us better see the world through their kaleidoscope of imperfect lenses.
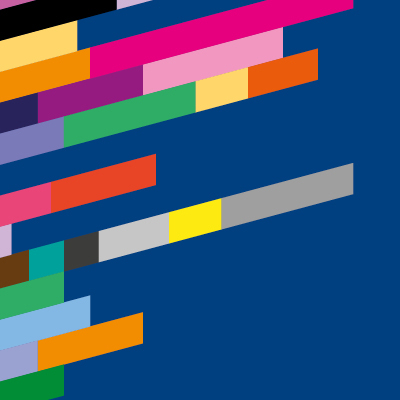
But, at least in the area of text analysis (AKA content analysis, or natural language processing), the old limits are crumbling, opening up a frontier of big social data that is fertile enough to support hundreds of research projects and next generation scientists.
The shift, like so many others, is coming thanks to advancements in data science technology. But it does not represent a total break with the past. For decades, social scientists have used expert document labeling tools, often called CAQDAS (Computer Assisted Qualitative Data Analysis Software), like AtlasTI, NVivo, and MaxQDA that allow a highly trained scholar to apply a custom set of labels to a few hundred documents. Initially built for labeling (AKA hand-coding) interview transcripts and meeting minutes, these tools are great for smaller projects. But training up a team to use them on a larger set of documents has always been a costly process of transferring expertise to research assistants and closely supervising their work to ensure its consistency. Lately, tools like Dedoose, DiscoverText, LightTag, and TagTog have re-factored this expert labeling approach into cloud-based software that is cheaper to deliver, update, and maintain—but their users still suffer the same bottleneck of expertise transfer. Even when they are applied to the simplest of labeling tasks, CAQDAS tools require the face-to-face training and oversight of a research team. In the university context, this management burden is grossly compounded by the high turnover among the transient student researcher workforce.
Crowds teaching machines
More recently, machine learning researchers have given social scientists hope. They have developed a number of new technologies able to harness the efforts of online workers to label data like images and video. The online workers go to a crowd work marketplace such as Amazon’s Mechanical Turk, where they are able to find brief ‘Human Intelligence Tasks’ they can perform through their Internet browser for a couple dollars per task. These crowd workers tend to be above average in education, and many do the work to earn supplemental household income. Already, they have completed billions of tasks applying highly accurate labels to images.
But the research community has been waiting a long time for a way to enlist the crowd in the much more difficult analysis of textual data like news articles, court proceedings, historical archives, and more. Computers–originally built to apply logical and mathematical operations on numeric, ordinal, and categorical data—still can’t faithfully understand human language. Computer scientists’ text mining (AKA natural language processing) approaches have been especially inadequate when it comes to applying, measuring, and testing the rich social theory scholars have built up over centuries. The clamoring for a high-scale, high-fidelity content labeling tool has only intensified since Benoit, Conway, and Laver published their landmark paper proving that crowds of independently working internet laborers could produce results as good as or better than experts.
Finally, the wait is over. My new company, Thusly Inc. is now providing the first and only full-service, end-to-end, online textual data labeling factory that can be custom-rigged for researchers’ use in a matter of several hours, and allow them to go as deep and as broad as they wish with their text labeling projects. No more compromises.
New, different, bigger, better
Notably, this new tool—called TagWorks—has not come from billion dollar tech companies like Amazon’s Mechanical Turk or Figure Eight. I imagined, designed, and developed it as a social scientist and data scientist intimately familiar with the headaches of managing annotation projects using the older, expert-dependent software. My vision of vast, powerful, richly labeled archives combined with my burning frustration as I tried to use other tools at high scale. I became obsessed with the goal of providing researchers with customizable annotation tools producing world-class, research-grade data in months, not years. So, while industry software developers are encouraged to “move fast and break things,” the TagWorks team and I have carefully ensured that researchers’ data will be accurate, reviewable, and reproducible—and articulate with even their most nuanced and elaborate theories about the social world.
TagWorks is one of a kind. Big tech companies like Figure Eight sometimes offer to build their customers annotation tools over the course of 4-6 months at great expense. (The folks at LightTag help you see the prohibitive cost of building even simple tools). But only TagWorks is optimized for efficient assembly line data flows and automated crowd task management. And only TagWorks provides interfaces specifically designed to guide non-expert crowd workers in the production of highly accurate data without face-to-face training.
Available now
For researchers who want high-quality data labels at a high-scale, the era of tradeoffs is over. You can label as many documents as you have with as many tags as you want. You can go deep. And you can go big. You can finally know and index everything important about your giant archive of documents. In a matter of months you could have the world’s most enriched archive in your discipline, or create the best labeled training data set producing the best text classification algorithm in your domain. To receive TagWorks’ free hybrid text analysis tips or schedule a free consultation to learn more about how you can advance the frontier in your field, email us at office@thusly.co or go to https://tag.works/get-started to get started.