When Do You Need to Trust a GenAI’s Input to Your Innovation Process?
In this post, co-authors Frank T. Piller, Tucker J. Marion, and Mahdi Srour reflect on the inspiration behind their research article, “Generative AI, Innovation, and Trust,” published in The Journal of Applied Behavioral Science.
In the rapidly evolving world of generative AI (GenAI), understanding how to integrate these technologies into the innovation processes effectively is crucial. In our research, we explore into how companies can harness the power of GenAI while maintaining trust in its outcomes.
Trust is a central concept when dealing with AI — we all experienced the power of ChatGPT when tasking it with knowledge-intensive tasks, but also its “hallucinations”—errors where AI generates misleading or incorrect information. In the context of innovation, this challenge is even more profound, as you want to create something new, hence do not easily know what the “truth” is.
Our collaboration began with a shared fascination for how AI can reshape innovation. Frank Piller, based at RWTH Aachen University in Germany, Tucker Marion and Mahdi Srour, who were researching at Northeastern University, connected over a startup called Ada IQ.
This Northeastern University spin-out is pioneering the integration of AI into every step of product development. Our joint interest in Ada IQ inspired us to explore how different types of AI models can be effectively employed across various stages of the innovation process.
The degree of trust required from GenAI outputs depends significantly on the phase of innovation. For instance, while GenAI’s hallucinations can be problematic in concept validation, they can foster creativity during an early ideation phase. Conversely, expert models trained on specific industry data are essential in later stages, requiring precise, domain-specific insights.
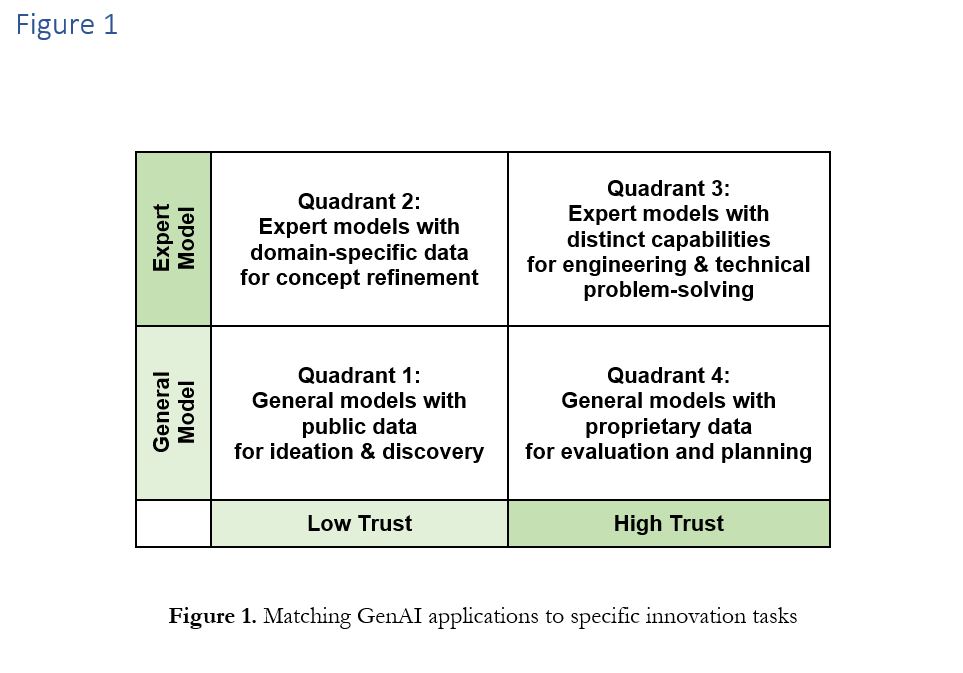
In our discussions, we quickly settled with three key questions for companies looking to integrate GenAI for innovation:
- the necessity of trust in AI outcomes,
- the choice between general and expert models,
- and the alignment of human capabilities with AI tools.
In our paper, we introduce a landscape to navigate the potential applications of GenAI in innovation management. This framework provides guidance on when to use general versus expert models and how to align AI tools with specific tasks and stages in the innovation process – always with the level of required trust in mind.
In consequence, companies need to invest not just in technology but also in developing human expertise to interpret and utilize AI outputs effectively. Here, the evolving capabilities of AI, including the rise of AI agents, provide interesting new opportunities and challenges.
In conclusion, our paper underscores the importance of a nuanced approach to GenAI integration, highlighting both the opportunities and challenges of this transformative technology. By using the landscape we developed, organizations can better navigate the complexities of GenAI, ultimately unlocking its full potential to drive innovation forward.